In this article we’ll discuss the essences of data transformations. This includes: how they tend to arise, their intended aims and the key steps and considerations needed to deliver a successful, deeply embedded transformation that has long lasting impact on the business.
In simple terms, data transformation projects consist of taking an organisation from a lesser to a greater degree of data maturity. In practice, this ought to mean starting from a holistic organisation-wide vision of data-centricity and conducting a gap analysis. This will involve a review of customer journeys, pain points and unmatched expectations, as well as a review of internal processes and data pipelines to identify bottlenecks and areas for optimisation.
Typically, such bottlenecks occur as a result of poor data management and data quality, a lack of data integration, low levels of analytical capabilities, or outdated technology infrastructure. As a result, data transformation projects, depending on the scale, tend to address one or multiple of the aforementioned. As we’ll argue, the more successful data transformations of any scale will take a more holistic view in addressing most if not all of them.
—
How do data transformation projects arise?
Large-scale data transformation projects are often prompted by several key factors:
1. Business strategy and growth: Leveraging data to enter new markets, launch new products or services, or drive operational efficiencies.
2. Technological advancements: Advancements in technology, such as the emergence of big data analytics, cloud computing, artificial intelligence (AI), and machine learning, can offer new possibilities for data collection, storage, processing, and analysis, which can significantly enhance business outcomes.
3. Competitive pressure: Organisations may feel the need to invest in similar initiatives as their competitors stay relevant in the market.
4. Regulatory compliance: Compliance with regulations around data governance or security may necessitate improvements in data management and governance practices.
5. Mergers and acquisitions: When organisations go through mergers, acquisitions, or restructuring, data transformation projects often become necessary. These projects aim to integrate disparate systems, consolidate data, and establish standardised processes across the newly formed or expanded organisation.
6. Data quality and accessibility challenges: organisations may realise that their existing data infrastructure and processes are inefficient, leading to data quality issues, limited accessibility, or siloed information.
7. Customer-centricity and personalisation: By leveraging data analytics and customer insights, organisations can tailor their offerings and interactions to meet individual customer needs.
—
Proactivity vs reactivity
The first potential pitfall occurs at the project initiation stage. A key question to ask here is the following: “Has the project been initiated as part of an effective data strategy?”.
In some cases, an organisation may lack a coherent data strategy. Strategic data imperatives may be implicit within the broader organisational or digital strategy, and a well-defined data strategy may not necessarily have been been specifically formulated.
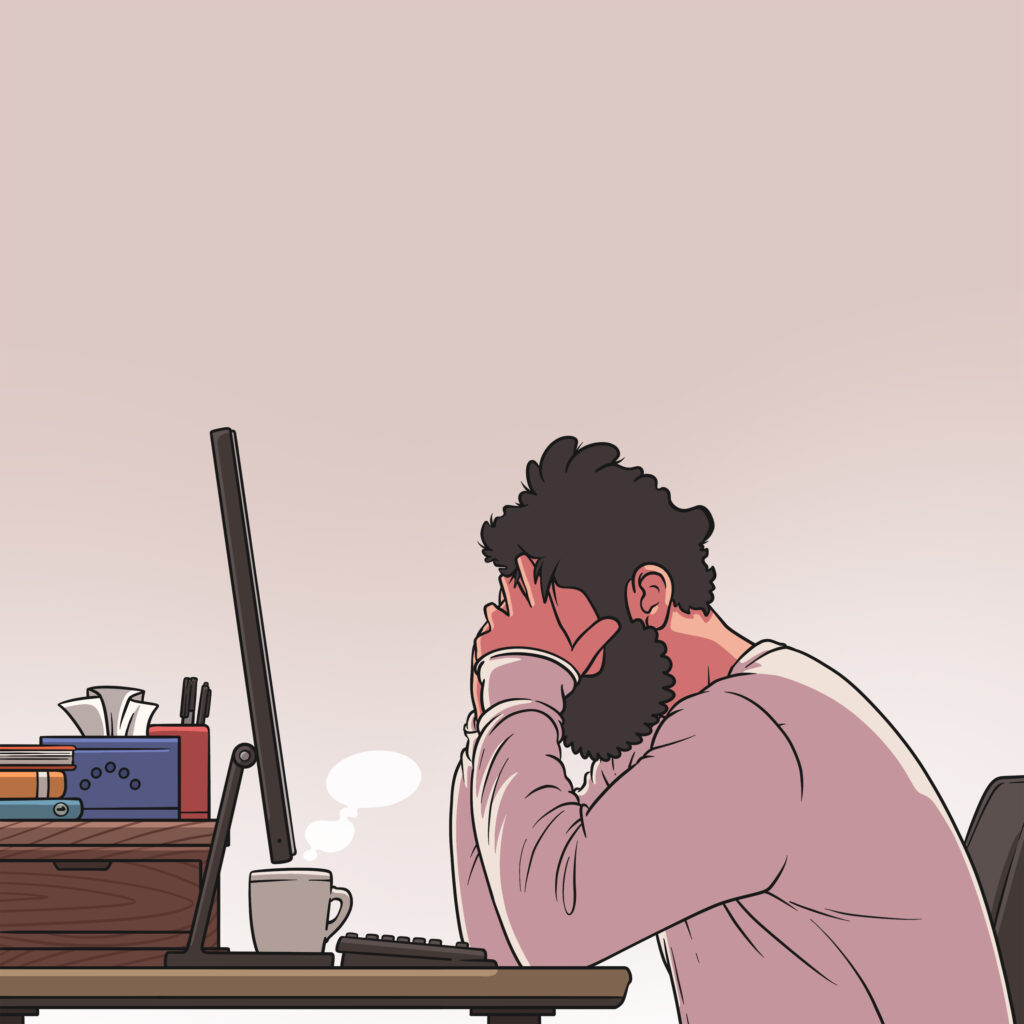
Such projects might be reactive in nature, responding to immediate data-related pain points or driven by specific business needs.
Reactivity poses significant challenges as it often results in disconnected projects that fail to align with strategic objectives and lack a holistic organisational perspective. This short-sighted approach neglects long-term implications, leading to a range of other issues, including the following:
- A lack of alignment within the business
- Fragmented and ad-hoc solutions
- Siloization
- Poor stakeholder management
- Time wasted on cross-departmental disagreements where projects are overlapping or co-dependent
- Duplication of efforts
- Difficulty with integration to business as usual
- Inefficient resource allocation causing delays, cost overruns, and inadequate project outcomes.
- Incomplete data coverage
- Limited scalability of solutions
The list is long, yet not exhaustive. A question arises: how do organisations specifically extract a data strategy from a broader organisational or digital strategy?
This, firstly, requires identifying the areas of the strategy which rely on or can benefit from effective data leverage, data governance and data-driven insights. This will help to inform a governance framework, data acquisition and integration requirements and articulation of requisite analytical capability. Capability is multifaceted; there are technical system-based capabilities and there are people capabilities.
Secondly, there needs to be methodology around cultural transformation i.e. how to ensure the organisational culture becomes more data-driven. Without this, most transformation projects will falter. Lastly, it requires establishing clear metrics for evaluating success.
—
Conducting a pre-mortem
Once a clear data strategy has been established, and the project aim is in pursuit of the aims of the strategy, the project conception stage is initiated. During this stage, it’s crucial to conduct a thorough sense check before committing to the project. This involves evaluating the feasibility, alignment with organisational goals, and potential risks and challenges. Common pitfalls at this stage include insufficient stakeholder engagement, unrealistic expectations, and inadequate consideration of resource requirements.
One effective approach is to conduct a pre-mortem, where the project team imagines a future scenario where the project has failed and identifies potential causes. This helps uncover hidden risks, gaps in planning, and areas that need further attention, allowing for proactive mitigation strategies and better project preparation. By conducting a comprehensive sense check and pre-mortem, organisations can increase the chances of project success and avoid costly mistakes.
—
Project execution
If the pre-mortem is conducted properly, many of the common pitfalls that haunt data transformation projects can be avoided. These can be broadly grouped into issues relating to the organisational culture, existing infrastructure and processes, and lack of digital capability within the organisation.
—
Organisational culture
Resistance to change is a common challenge in data transformation projects. Overcoming resistance requires effective change management strategies, clear communication about the vision and goals of the transformation, and involving employees in the process.
Data transformation projects also require cross-functional collaboration and the breaking down of organisational silos, which can be achieved by creating channels for knowledge and idea sharing, and establishing multidisciplinary teams or task forces to work on transformation initiatives.
—
Existing infrastructure and processes
Legacy systems and infrastructure: Outdated legacy systems can pose a significant barrier to transformation. It can require significant investments and careful planning to modernise the tech infrastructure. It’s important to prioritise investments in upgrading or replacing outdated systems, but there are also solutions which can be leveraged in the transition to new infrastructures, such as cloud-based solutions and the leveraging APIs to integrate new technologies with existing systems.
—
Digital capability
Data transformations often demand new skill sets and expertise. It’s important to assess the skills needed for digital transformation and identify any skill gaps within the organisation. However, upskilling does not necessarily mean rolling out mass training programmes. Good upskilling is time-efficient and tailored to skill levels and context (not just one size fits all). Whilst people should be made aware of the project and made aware of the capabilities they’ll need to develop in advance, the actual upskilling should be delivered at the point of need. That way it can be immediately put into use, and be connected to concrete outcomes.
—
Project handover and integration to business as usual
The importance of handover is often neglected. Projects are by definition impermanent, and yet they exist as part of a chain. That’s because transformation is a consistently evolving entity. As a result, it’s imperative that they can smoothly be handed over to operational business units. Care needs to be taken to put measures in place which aid the transition. Check in points also need to be created in the initial stages of handover to ensure teething issues and resistance are properly resolved. When this doesn’t happen, systems and processes are often discarded or fall out of use, rendering their conception pointless.
—
In summary
Data transformations originate for a number of reasons, but there are common pitfalls inherent to them all. However, by aligning to a clear data strategy, identifying potential pitfalls ahead of time, addressing organisational change from a holistic perspective, and focusing on smooth integration to business as usual, organisations can ensure successful data transformation projects that deliver long term business value.
Begin your data transformation by hiring the right people. Let PL Talents help you with that.