The rapid advancements in AI tools and machine learning have revolutionised various industries, offering powerful solutions to complex problems. As developers incorporate AI-powered tools into their work, it is essential to recognise the ethical responsibilities that come with this technology. While AI holds tremendous potential for positive impact, it also carries inherent risks. These risks can lead to harm if not managed responsibly.
This article delves into the ethical considerations that developers must be aware of when implementing AI tools and machine learning in a commercial setting. By making conscious decisions during the development and deployment of AI tools, developers can help ensure that their innovations promote fairness, environmental awareness, privacy, and inclusivity.
—
Information leaks and privacy
One major ethical challenge for developers is the potential for agents, malicious or otherwise, to identify sensitive information from the training data. This can happen purposefully through reverse engineering, or even accidentally by specific prompts. And while anonymising training data is a common privacy protection measure, achieving complete anonymity is not always feasible. Traditional anonymisation methods, like removing PII, may not be enough to ensure complete privacy protection.
To overcome these challenges, developers should consider advanced privacy-preserving techniques, such as the below:
- Differential Privacy
This is a technique that adds controlled randomness to data before training machine learning models, preventing specific data points from being reverse-engineered.
- Federated Learning
This is a decentralised approach where models are trained on individual devices. Only model updates are shared with a central server, preserving data privacy and reducing the risk of data exposure.
- Secure Multi-Party Computation
This allows multiple parties to jointly compute a function on their private inputs without sharing the raw data, ensuring data privacy during collaborative machine learning tasks.
Comprehensive risk assessments and regular audits can help identify vulnerabilities and monitor model behaviour. Clear communication and consent mechanisms should be established to ensure users are informed about how their data will be used. By adopting responsible AI practices, developers can build trustworthy AI solutions that safeguard user privacy while maintaining model effectiveness.
Discrimination and inequality
AI tools, particularly machine learning models, can inadvertently perpetuate biases and generate exclusionary or toxic content. For instance, a chatbot designed to assist customers might unknowingly respond to certain queries with biased or offensive language, reflecting the biases present in its training data. Facial recognition systems built on biased datasets may perform poorly on individuals from under-represented communities, leading to unequal treatment. To tackle this issue, developers should carefully curate training data to minimise biased or harmful content, and ensure adequately representation of different demographics.
Security vulnerabilities
Implementing machine learning models, especially those employing complex architectures like GANs (Generative Adversarial Networks) and deep reinforcement learning, can introduce security vulnerabilities. Adversarial attacks are a significant concern in AI, where malicious actors deliberately manipulate the model’s input to cause misclassification or false outputs. For instance, an autonomous vehicle controlled by a deep reinforcement learning system could be misled by adversarial inputs, leading to dangerous driving behaviour. Ensuring robustness against such attacks and designing models with adversarial robustness in mind is crucial for maintaining the security and reliability of AI applications.
Misinformation risks
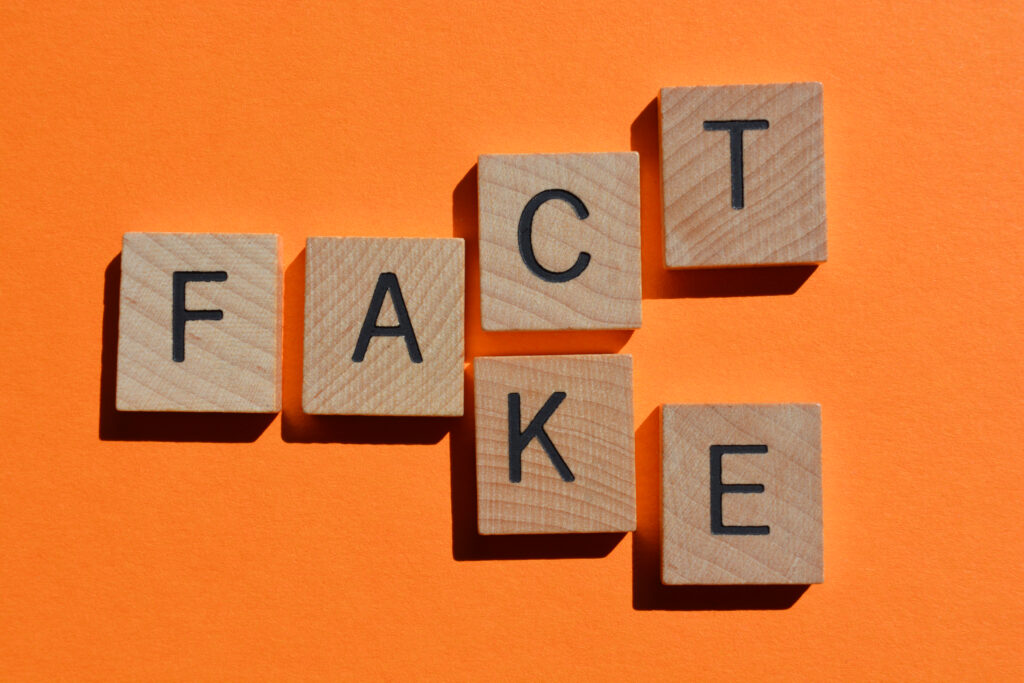
AI-powered tools can inadvertently propagate false or misleading information. For instance, a language generation model might generate misleading news articles or social media posts that spread misinformation. Developers must take measures to minimise misinformation risks and promote reliable AI outputs.
To combat misinformation, developers can integrate fact-checking algorithms into AI systems to verify the accuracy of generated content. Enhancing AI models’ contextual understanding capabilities can reduce the risk of generating misleading or false information. For example, cross-referencing AI-generated content with verified sources can help identify and flag potential misinformation.
Risks associated with human-computer interaction
AI tools designed for human-computer interaction, such as chatbots, virtual assistants, and social robots, are becoming increasingly prevalent in various domains. While these tools offer great potential for improving user experiences and providing valuable services, they can also inadvertently cause harm if not handled responsibly.
One of the primary ethical concerns with AI tools that interface with users is the risk of over-anthropomorphisation, whereby humans infer human characteristics from the AI they’re interfacing with. It might sound overblown, but it can act in pernicious ways, whereby individuals overly trust the outputs of an AI tool or rely on them for emotional support. For instance, an AI-powered chatbot designed to sell products may employ psychological tactics to encourage impulsive buying, exploiting users’ emotional vulnerabilities.
Users who overly trust AI may blindly follow its recommendations, even when they are incorrect or harmful. For instance, trusting an AI medical diagnostic tool without questioning its output could lead to misdiagnosis or delayed medical treatment.
To address these risks, developers of AI tools for human-computer interaction must prioritise transparency and clear communication with users. Users should be informed about the limitations of the AI system with explicit disclaimers or non-human-like visual representations to reinforce the fact that AI tools are machines, not humans. Implementing error reporting and feedback mechanisms allows users to report harmful outputs, enabling continuous model improvement and risk mitigation.
Environmental risks
Environmental risks associated with AI implementation arise primarily from the energy-intensive nature of training and deploying large-scale AI models. These processes can lead to increased carbon emissions and contribute to environmental resource depletion, such as increased demand for electricity and cooling systems in data centres.
One of the key considerations for developers when implementing AI models is the choice of infrastructure and hosting services. Developers can try and opt for cloud services or data centres that utilise renewable energy sources.
In addition to the choice of hosting services, developers can also explore techniques to optimise the energy efficiency of AI models during training and inference. Techniques like model pruning, quantisation, and efficient hardware design can help reduce the computational requirements, leading to lower energy consumption and environmental impact.
Moreover, developers can adopt best practices for AI model development that focus on data efficiency. Training large-scale AI models typically involves extensive data collection and processing, which can consume significant resources. By using data augmentation, transfer learning, and other techniques, developers can achieve comparable performance with less data, minimising the environmental impact of data-intensive processes.
In conclusion
Developers hold a significant responsibility in shaping the ethical implementation of AI tools and machine learning models. Collaboration between developers, researchers, policymakers, and users is crucial to address ethical risks effectively. It is also essential to foster continuous improvement in AI technologies.
It is through collective efforts and a commitment to responsible AI practices that developers can shape an AI-powered future… Which aligns with ethical principles, respects individual rights, and benefits society as a whole. As AI continues to evolve and permeate various aspects of our lives, it is imperative to prioritise ethics, accountability, and social well-being in the development and deployment of AI tools and machine learning models.
—
If you are looking to build ground-breaking AI solutions, you need the right team. Contact a PL Talents expert today, and we will connect you with the top candidates.