Data teams have evolved significantly in the past decade due to technological advancements, data availability, and recognition of data-driven decision-making. We’ve seen the growth of data democratisation, data engineering and the integration of sophisticated machine learning modelling in organisations of every size and sector.
This therefore begs the question, as the landscape of data analytics continues to evolve at a rapid pace, how do we build data teams that can evolve alongside it?
To build a successful and adaptable data team, several crucial elements must be considered: recruiting and retaining talent, fostering a culture of continuous learning and development, creating an appropriate, flexible team structure, and establishing a strong alignment between the organisation’s goals and the data team’s key performance indicators (KPIs).
—
Recruitment
Data roles are difficult to recruit for, but there are a couple of key considerations to ensure the right people are recruited into the right roles, so as to avoid low utilisation of skills, employee dissatisfaction and high churn rates.
1) Understanding data analytics
Organisations often face challenges in understanding the intricacies of data analytics and determining the appropriate roles for their data teams. Without a clear grasp of their specific data problems, organisations struggle to define these roles accurately. To avoid problems later down the line, recruiters need to avoid ambiguous job descriptions and instead outline the precise skills required. It’s important to balance hiring for the immediate needs of the organisation vs the far-long term aspirations. This is important for managing the expectations of new hires e.g. not hiring for interesting ML projects if data professionals are likely to write SQL queries all day.
2) Recruiting rigorously whilst identifying diverse talent
There’s a wealth of untapped talent which hasn’t been through the traditional routes, but it requires more creativity and awareness to identify it. With the rise of micro-credentials, online learning and boot-camps, it can be difficult to vet ability, particularly as there’s such tough competition for data professionals, which makes long winded recruitment processes undesirable. Equally, the titles within data analytics, due to the trend-worthiness of the field, have become nebulous and ill-defined over time.
It’s therefore important to look beyond job titles and instead assess capabilities via tangible projects. What’s more, there needs to be a focus on non technical ability such as curiosity, collaboration and adaptability.
—
Structuring the data team
The appropriate structure of a data team is influenced by an organisations size, structure, data architecture and data maturity.
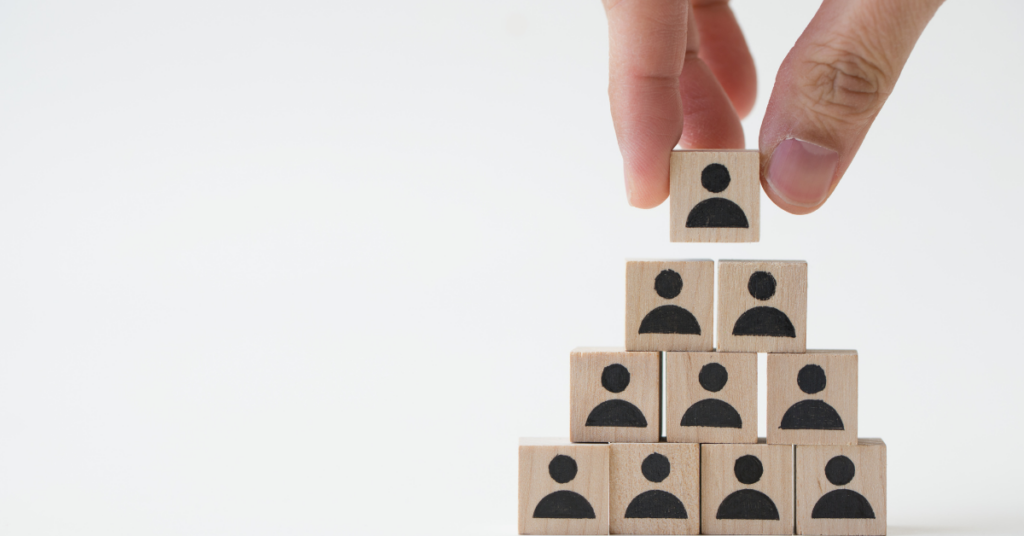
Larger organisations often require a more complex data team structure to handle the diverse data needs across different departments and business units, whereas smaller organisations may have leaner data teams with individuals wearing multiple hats and handling a broader range of responsibilities.
Teams may be centralised to ensure consistency in data management and analysis practices or instead organisations may decide on decentralised or hybrid data team structures, with data professionals embedded within different departments or business units to support their specific needs.
Organisations with a higher level of data maturity, including robust data governance practices and established data pipelines, may require specialised roles focused on data quality, data governance, or data operations within the data team.
In any case, it may be worth investing in more flexible or agile team structures, so as to ensure the team can evolve and adapt over time. Agile methodologies allow the data team to break down complex projects into smaller, manageable tasks. The iterative nature of agile methodologies also encourages the team to regularly reassess priorities, making it easier to incorporate new data sources, technologies, or evolving business needs into their workflow.
—
Executive buy-in
One of the factors that contributes to whether or not a data team is truly future-proof is if it grows with the business, as opposed to stagnating as a result of low executive buy in and poor investment.
Much like any other function, there needs to be a clear scope for the data function, regardless of how the data capabilities are structured within an organisation. This means a dynamic alignment between the organisational strategy and data strategy, ensuring the organisation as a whole is data-driven and the role of data analytics isn’t silo-ised or underutilised.
Occasionally, we observe that organisations expect too much too soon with regards to their own data maturity. Aspiration needs to be met with an assessment of the current state, including data architecture, accessibility and analytical capabilities. This can then help with appropriate resource allocation, investment in infrastructure and data literacy.
—
Organisational culture
Creating a culture of experimentation and learning is crucial for the longevity of teams. Organisations need to promote a safe environment for experimentation and continuous learning.
Providing various opportunities for continued learning is also vital, this can be achieved by allowing periods of ‘buffer time’ between projects for learning and reflection, promoting team knowledge-sharing, allowing personal projects, and facilitating collaboration with other business functions. On-the-job learning and mentorship also contribute to expanding team members’ technical and non-technical skills.
By implementing these practices, organisations can establish a genuinely innovation-focused culture that promotes collaboration, experimentation, learning, and continuous improvement within their data teams.
—
In conclusion
To build a data team that can thrive in the long term, there must be a focus on the following:
- understanding the organisation’s data needs, defining roles accurately, and identifying diverse talent beyond traditional routes.
- tailoring team structure to the organisation’s size, structure, and data maturity, with flexibility and adaptability as key considerations.
- ensuring investment at the senior-leadership level, to ensure the team’s growth aligns with the organisation’s strategy
- fostering a data-driven culture across the entire organization, as well as a culture of experimentation and continuous learning
By implementing these practices, organisations can establish data teams that are well-equipped to navigate the rapidly changing landscape of data analytics.
If you are looking for your next dream data team, we have the expertise and the candidates. Get in touch with us today.